We welcome students from a variety of backgrounds in four graduate programs and offer many research opportunities.
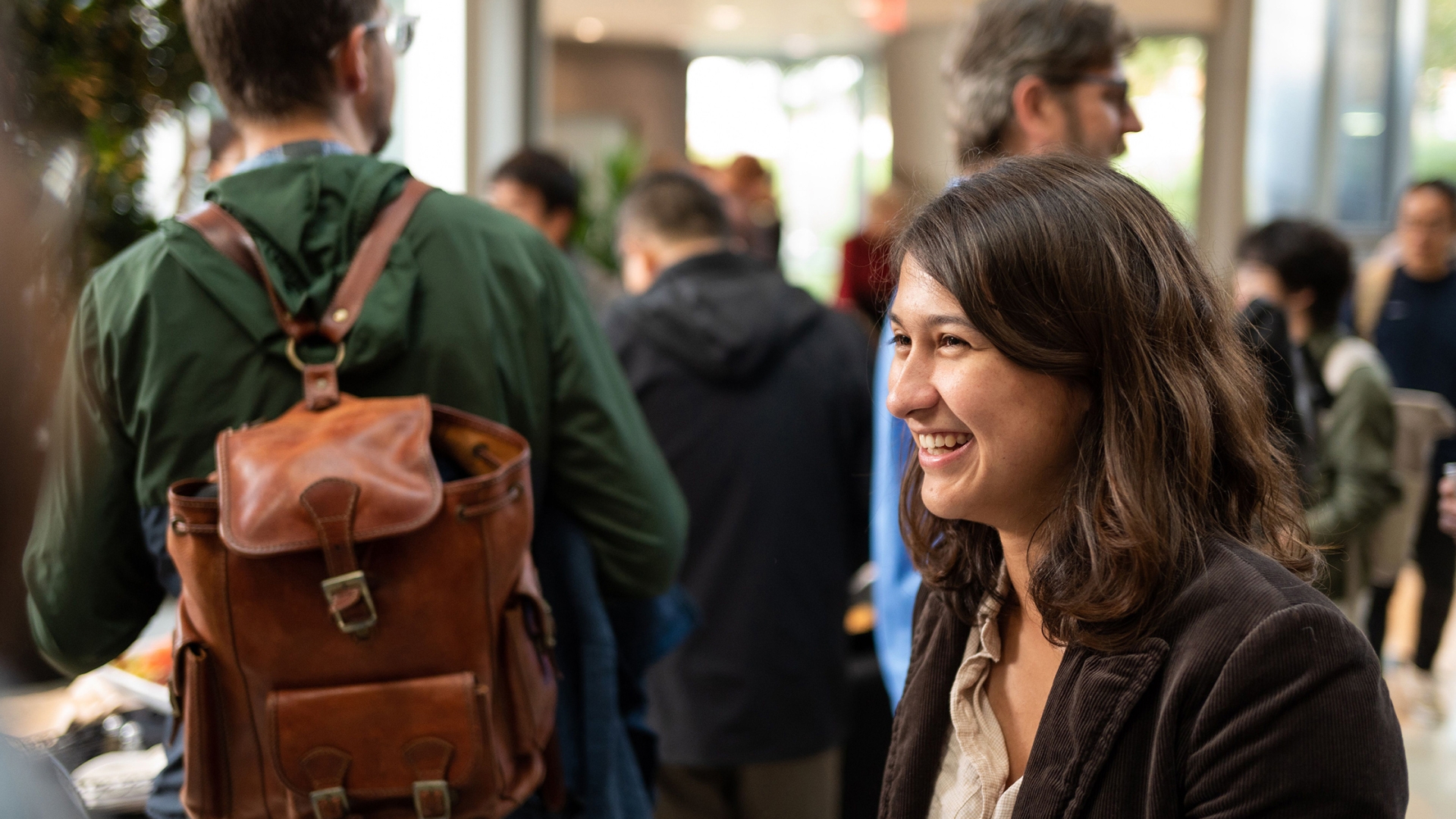
The study of computational medicine and bioinformatics prepares students for careers in biomedical research in academia or in industry. We pursue world-class interdisciplinary research and teach how to develop and apply leading-edge computational methods and tools.
100 Washtenaw Avenue
Ann Arbor, MI 48109-2218
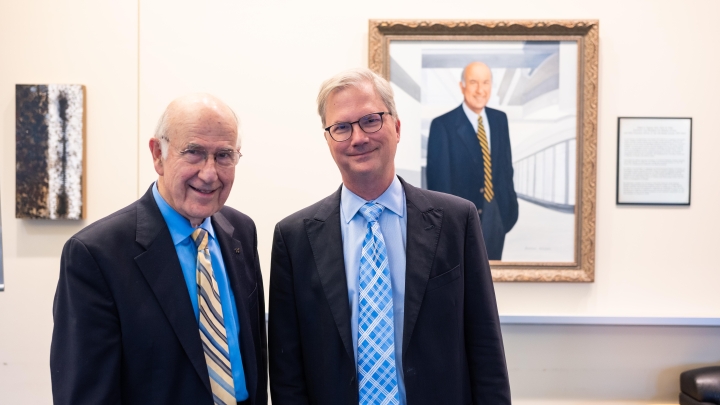
Our interdisciplinary center is the home of innovative research and cross-campus collaboration. Here, experts from across schools and departments work together to advance biomedical knowledge and its therapeutic applications.
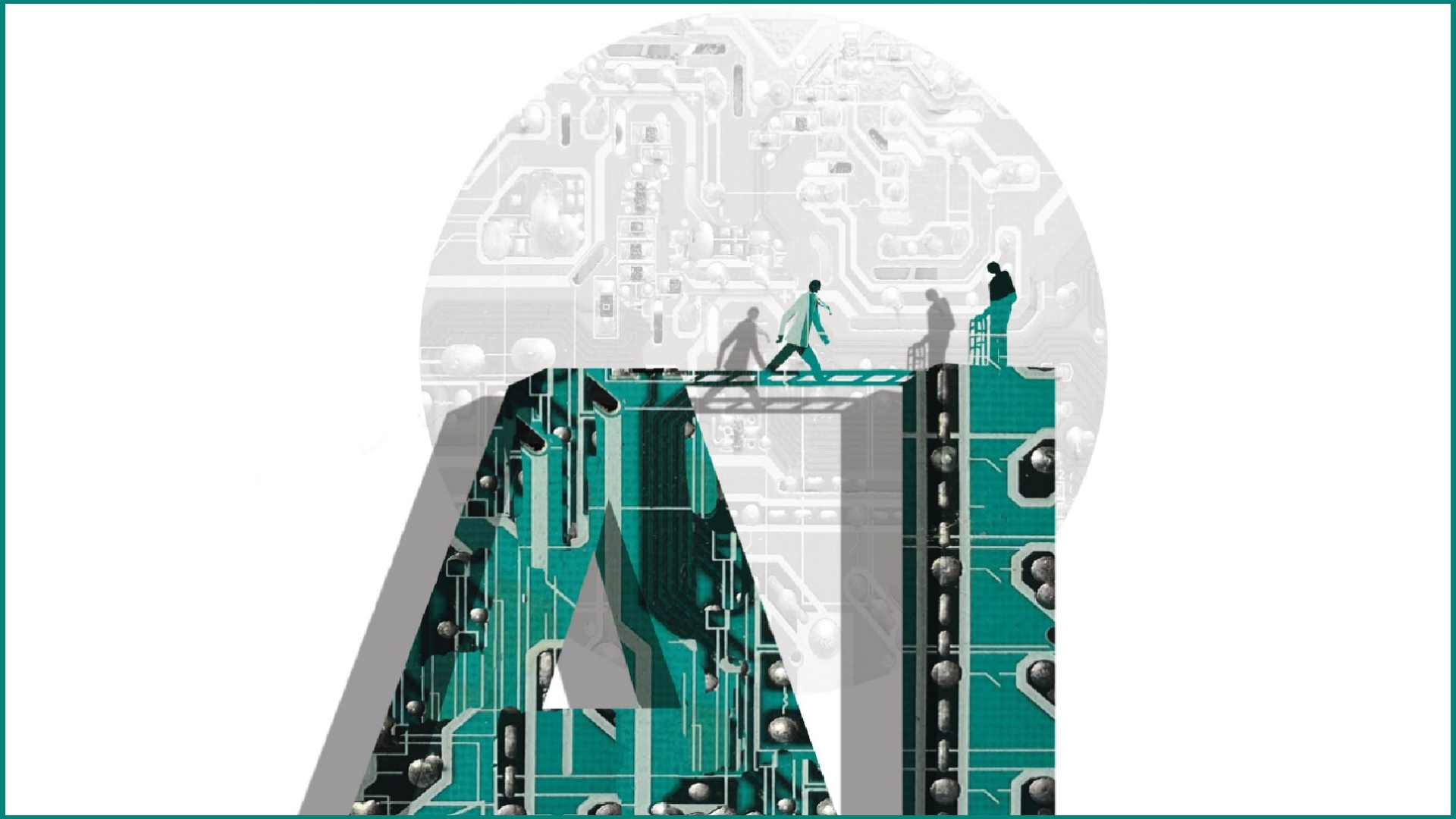
Learn more about the Computational Medicine and Bioinformatics Department in this short video.
Our department offers four degrees: PhD, Master's, Accelerated Master's and Dual Degree. It is supported in part by two NIH Training Grants.
Help our trainees become the innovators of the cures and technologies of tomorrow.
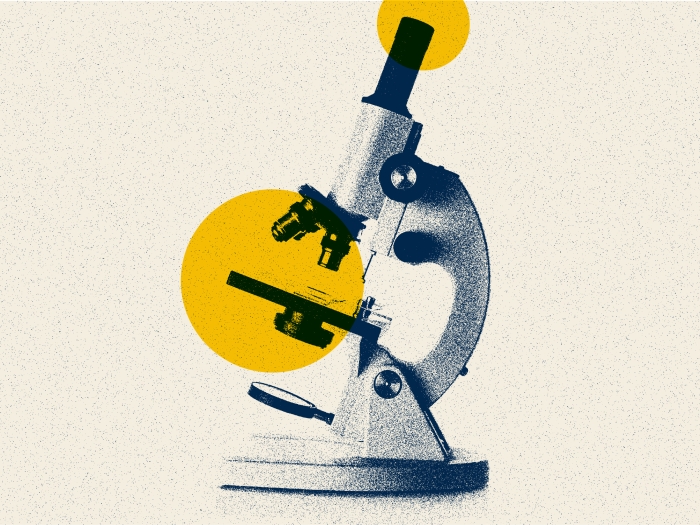
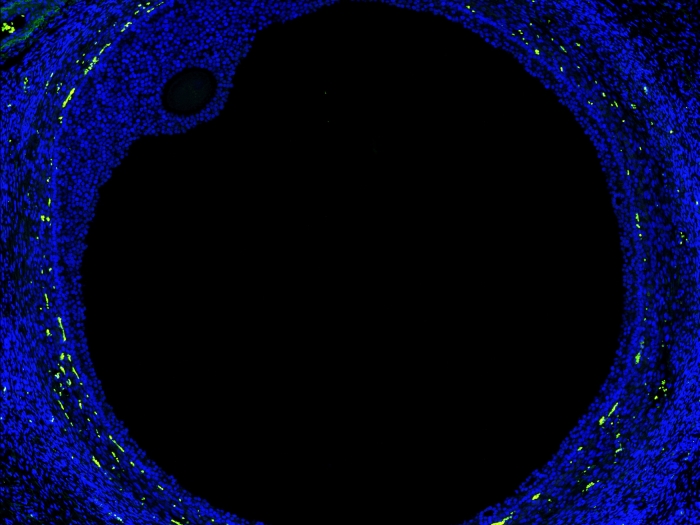
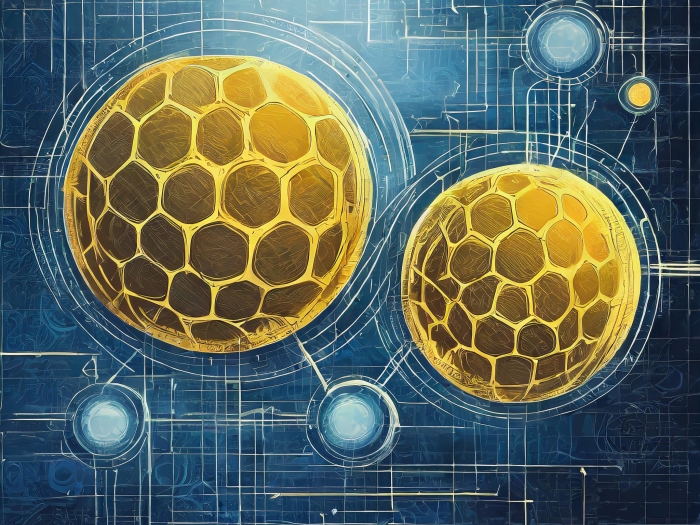
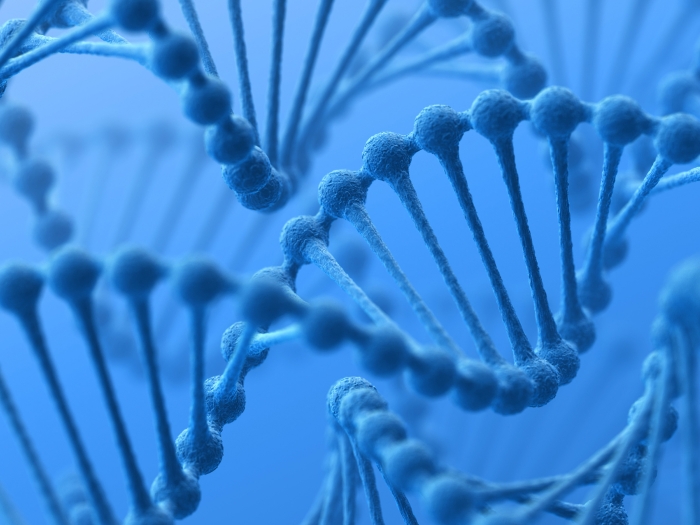
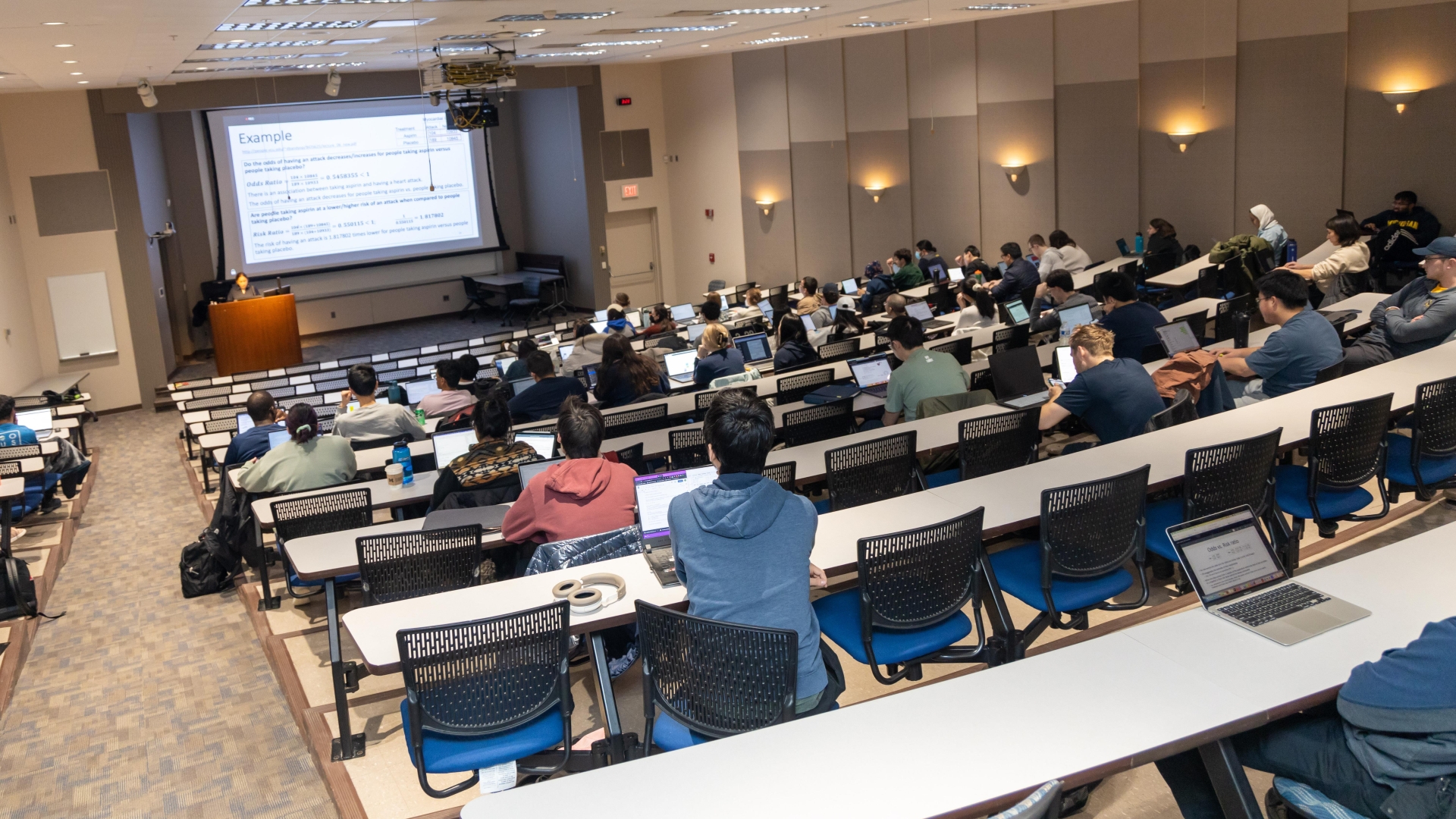